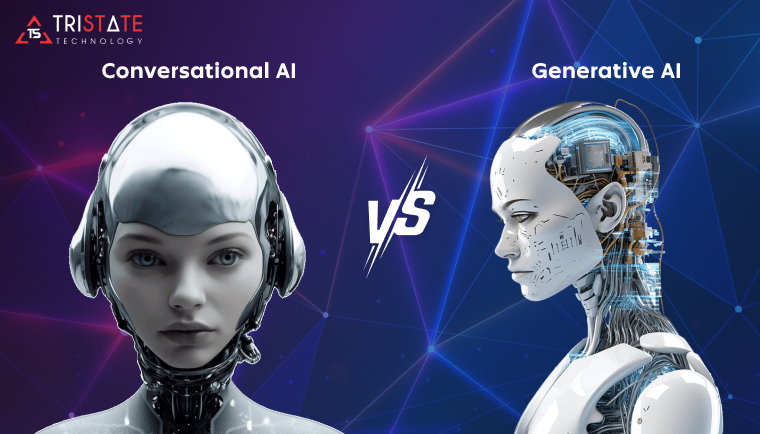
Do you have any idea how rapidly AI technologies are booming in the business world? As per research by IBM, over 42% of IT experts in large-scale business organizations have already incorporated artificial intelligence into their operational tasks. And, the rest, 40%, are enthusiastically looking for opportunities to achieve this. So, clearly, AI has become a mainstream technology for today’s modern-age business.
Financial services companies are highly reliant on implementing AI. Similarly, 37% of total IT experts in the telecommunications business also confirm that their companies have adopted AI solutions. This shows a growing trend of AI adoption across various industries.
With time, AI technology has become vast and has become an extraordinary type of technology that meets various industrial needs. Conversational AI and generative AI are currently trending across business sectors globally.
The conversational AI market size is to reach USD 49.9 billion, and the Gen AI market is to achieve USD 136.7 billion by 2030 future.
All these statistical figures state that both conversational and generative AI technologies are playing a leading role in assisting businesses in various activities.
Move ahead to get a deeper and clearer insight into Conversational AI vs Generative AI, including their key differences and use cases. This guide will deliver you an in-depth understanding of choosing the right AI technology for your business.
Table of Contents
Overview of Conversational AI
Conversational AI is an artificial intelligence model that delivers human-like conversation through the use of ML and NLU to let computerized devices process human language. So, the Conversational AI model delivers hyper-personalized and more intelligent conversations.
Applications of conversational AI are now global; they range from virtual assistants such as Alexa to customer service chatbots and automated support models. The AI model can handle various activities like answering questions, giving necessary recommendations, and solving issues, all while learning from past interactions.
As these technologies started evolving, conversational AI became more sophisticated, offering greater personalization and more accurate and meaningful conversations. This advancement is transforming different industries.
Overview of Generative AI
Gen AI technology is a type of artificial intelligence model. Want to know what isGenerative AI?in detail. It aids in generating different content types, such as text content, multimedia content, and codes. It employs machine learning models in order to deliver creative outputs based on patterns obtained from vast datasets.
Using Generative AI, businesses get assistance in writing documents, designing and delivering high-quality content, problem-solving, and simulating real-world environments and conversations. The technology can generate all these in seconds.
Surprisingly, Generative AI is not a recent innovation, as it first appeared during the 1960s with the advent of chatbots. However, it wasn’t until 2014, with the development of generative adversarial networks (GANs), that the technology gained immense popularity due to its ability to produce convincingly realistic multimedia content. Since then, GANs have moved on to advancing generative AI’s capabilities.
Various AI algorithms then return new content in response to the prompt. Generative AI starts working with a command or prompt. It can be anything simple text, media input, design input, or anything. However, you need to ensure that the system is capable of processing all types of inputs.
Different AI algorithms analyze the inputs and generate the required response. This output is usually creative text content, solutions, or multimedia output such as audio as humans or images. Consequently, Gen AI delivers a wide range of creative and practical outputs.
The older versions of gen AI require users to submit data input via an API or a complicated process. During this period, developers have to learn specialized tools and write codes in programming languages like Python. As a result, the process was more challenging and less accessible for many.
But now, modern-age developers are capable of creating improved user experiences using generative AI, allowing users to make requests in simple language. After receiving an initial response, developers can customize the results from the feedback on different elements such as format, design, tone, etc. This approach makes it easier to generate content that aligns with user preferences.
Distinguish Between Conversational AI and Generative AI
Here is the Difference between Conversational AI and Generative AIin terms of features;
1. Core Functionality
Conversational AI enables intuitive and interactive communication with users by employing natural language processing. Moreover, it lets users interact and engage in dialogue with machines in a human-like manner.
On the other hand, Generative AI is known for producing original and unique content by analyzing patterns in input data. It results in new outputs based on the information it has learned from various sources on the Internet.
2. Applications
The Conversational AI and Generative AI differ completely in terms of applications. Conversational AI is primarily used to provide quick customer assistance, addressing their queries and solving their issues immediately. The technology is used as a virtual assistant to assist users in their product purchase journey. Moreover, they are also employed as educational bots to provide informational data and educate users on different concerns.
GenAIis used to create unique content, including creative designs and media outputs. Furthermore, it supports data analysis and simulations. This technology enhances various fields by generating new and valuable outputs based on data patterns.
3. Technology Usage
Conversational AI uses smart technologies such as NLP and ML tech to identify and generate answers to user inputs. It employs these technologies to interpret language and generate appropriate replies. Through this process, Conversational AI can interact with thoughtful conversations with users.
Generative AI utilizes deep learning networks to generate text and media content. It employs these networks to analyze data patterns and generate new content. With this, Generative AI delivers a wide range of creative outputs.
4. Impact on Business
Conversational AI aids digital-age businesses by taking their user engagement to an advanced level with hassle-free interactions. The technology uses its power to automate customer interactions with rapid and precise responses. This technology helps businesses enhance communication with users while reducing manual effort.
Generative AI Development Services fuels innovation by automating the unique procedure of developing content. It enables the creation of new content, streamlining business tasks. This AI technology assists in pushing the boundaries of creativity and productivity and thinking beyond for various industries.
A Clear Overview of Benefits and Drawbacks: Generative AI vs Conversational AI
Let’s understand the Benefits of Generative AI and its limitations;
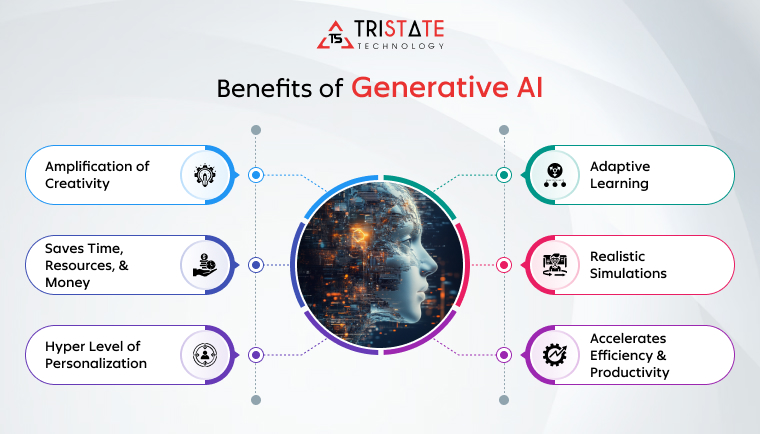
Amplification of Creativity
Generative AI is programmed to deliver highly creative content. For instance, it has the ability to generate compelling advertisements and visually attractive media content using innovative technologies. It seamlessly automates the creative content generation process with the necessity of manual efforts.
Saves Time, Resources, and Money
Generative AI saves your precious time and capital by automating tasks. In the future, you will witness a decrease in your overhead cost using Gen AI. For example, the construction industry can employ AI algorithms to create complex building designs. Thus, it will speed up the complete design process.
Hyper Level of Personalization
Businesses can make their user experience highly personalized with the help of Gen AI. The technology analyzes data to create personalized product recommendations and service demonstrations. In addition, Gen AI personalized customer support, enabling e-commerce businesses to use dynamic and personalized voices for voice automation. This makes interactions more natural and human-like.
Accelerates Efficiency and Productivity
Generative AI supports businesses in automating simple to complex tasks, improving workflow efficiency and resulting in great productivity. In the manufacturing sector, Gen AI creates optimized production schedules and facilitates the reduction of waste discharge. In contact centers, Gen AI supports agents by summarizing tickets and suggesting context-based responses.
Realistic Simulations
Gen AI creates realistic simulations to utilize for education and research purposes. AI simulators are used in the automotive sector to replicate driving scenarios for safer, more autonomous vehicle testing. This AI technology brings in testing accuracy and boosts safety.
Adaptive Learning
Generative AI systems have the capability to understand and adapt by processing feedback and new input. This ability helps the models improve and create outputs that better meet user preferences. In the education sector, generative AI personalized learning materials and methodologies to suit each student’s learning style, improving educational outcomes.
Limitations of Generative AI
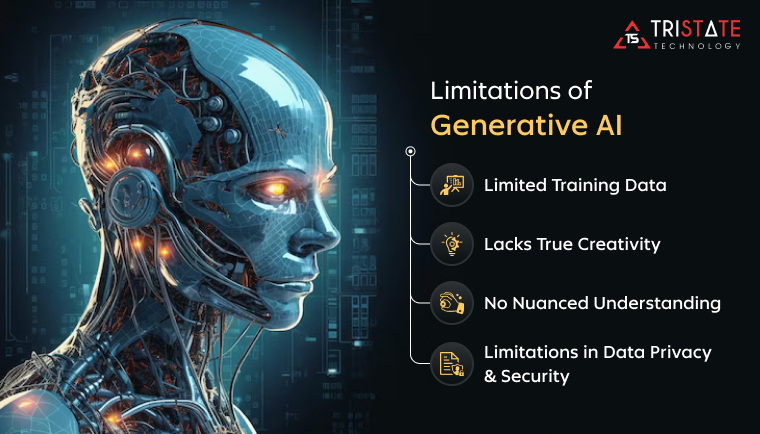
Limited Training Data
Generative AI models completely depend on the data they are programmed or trained on, which is often limited. Research shows that if the training data contains factual errors or biases, the AI’s generated content will reflect similar issues, such as reinforcing historical gender bias content in news articles without any research.
Lacks True Creativity
No doubt, Generative AI can quickly produce any type of content or code within seconds, but it doesn’t create entirely from scratch, and it actually lacks creativity. A study revealed that these AI models remix training data by recognizing patterns, yet they lacked true creativity and understanding of underlying concepts.
No Nuanced Understanding
Generative AI often has trouble studying human language, such as emotions and humor. Research shows that these models struggle with humor because human humor relies on context, unpredicted twists, and sudden emotional change, which current AI models find difficult to interpret.
Limitations in Data Privacy and Security
Training generative AI apps demand access to data, leading to potential breaches and violations. These models do not guarantee that your confidential data will be safer.
Overview of Benefits of Conversational AI with their limitations;
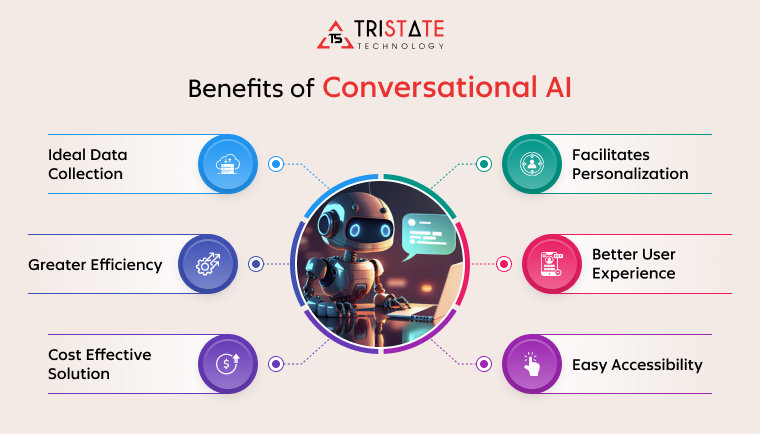
Ideal Data Collection
Businesses always look forward to constantly improving their marketing methodologies and sales to compete against emerging competitors. For this, they need to generate leads by fetching customer data.
You can simplify the procedure with Conversational AI as it can conduct optimal data collection. It has the power to monitor and keep track of customer conversations and behavior changes. With this, the Gen AI model can fetch relevant data on consumers’ interests.
Greater Efficiency
Conversational AI can manage multiple works without being dependent on humans. This frees up employees in business organizations to focus on other aspects of personalized customer satisfaction and management processes, boosting scalability by reducing time spent on repetitive tasks.
Cost Effective Solution
A conversational AI model runs autonomously with minimal or zero supervision, significantly reducing overhead operating costs. In a telecommunication office, this AI technology tracks calls, evaluates user engagement, and manages huge call volumes, ultimately driving the revenue rate.
Easy Accessibility
Improved user availability boosts their experience from the very beginning. With conversational AI being omnichannel, your users can well interact through their preferred method of communication such as call or text.
In addition, they will instantly get responses to their queries, avoiding long queues of phone calls.
Better User Experience
Conversational AI is comprehensively programmed to personalize and improve customer experience through easy and top-notch communication, as well as solve problems in one go.
Conversational AI can take your user experience to the next level with its power to personalize interactions with tailored responses and specific information.
Facilitates Personalization
Conversational AI, at times, employs machine learning to deliver a fully personalized experience for each user based on relevant data such as search history, current location, and usual preference. As it gathers and learns from customer interactions over time, the AI can provide highly relevant information and promptly suggest the next actions tailored to the user’s necessity.
Limitations of Conversational AI:
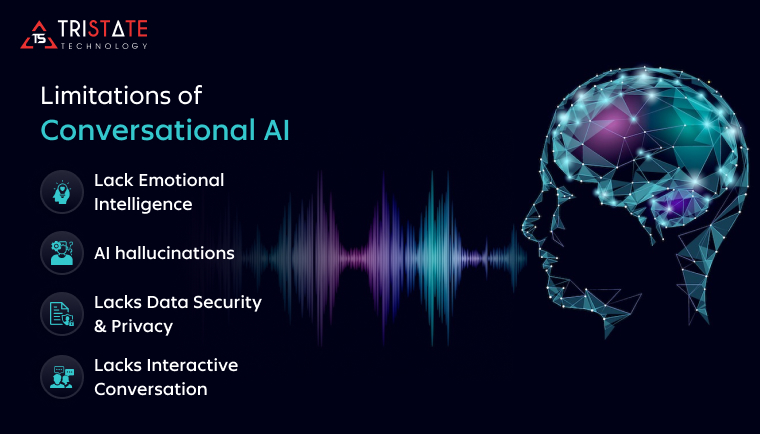
Lack Emotional Intelligence
Although conversational AI has the ability to simulate human interactions, it struggles hard to replicate true human-like emotional intelligence and empathy. This lack of emotional understanding limits the models’ ability to offer a meaningful user experience. Moreover, it cannot fully respond to customers’ mixed feelings, reactions, and concerns.
AI hallucinations
AI hallucinations occur when conversational AI models such as chatbots at times deliver inaccurate, insensitive, or misinformation to user questions. This happens not because the model intends to deceive but due to limitations in its programmed data as well as complications in language processing.
Lacks Data Security and Privacy
Conversational AI often lacks robust data security and privacy measures, making sensitive customer information vulnerable. This gap in data protection can put businesses at risk, exposing them to data theft and misuse. Without strong safeguards, users may not share personal details and trust in the model.
Lacks Interactive Conversation
Conversational AI often lacks the ability to maintain truly interactive conversations. It can struggle with context, tone, and adapting to complex or unpredictable user inputs. As a result, interactions can feel robotic and limited, reducing the overall user experience.
Latest Technologies Empowering Conversational AI
Advanced NLU
Conversational AI is currently improving its features to understand human language through advanced natural language understanding. It can now handle multiple user intents with great accuracy.
This advancement in NLU resulted in fewer misinterpretations during conversations. Also, it enhances the model’s ability to manage complex requests accurately.
Machine Learning
Machine learning (ML) powers up conversational AI models by propelling systems to study from input and improve output. ML algorithms analyze patterns in user interactions, helping AI understand language, context, and intent.
This allows conversational AI to generate more relevant responses, adapt to different scenarios, and increase user engagement. You can know more about it through data science & data analytics services with AI.
Voice and Multimodal
Conversational AI models are now integrated with advanced voice recognition and visual interfaces. Voice AI incorporation advances clear and more natural conversations; on the other hand, multimodal integration combines all content types, such as text, voice, and visual, to create richer conversations.
In addition, conversational IVR, supported by advanced NLU, replaces traditional calling systems to let users speak with automated systems.
Advanced Technologies in Generative AI
Natural Language Processing (NLP)
NLP enables generative AI to evaluate and deliver human-like text. It helps AI systems process large amounts of text data, recognize context, and produce coherent and contextually appropriate responses, making it essential for tasks like text generation, translation, and summarization.
Deep Learning
Deep learning is employed to identify complex patterns and bonds in large datasets. It is the foundation for generative AI, enabling systems to create realistic content such as images, music, and text by learning from massive amounts of data through layers of processing.
GANs
GANs are a part of the deep learning framework to deliver genuine content. Together, they help AI improve and generate high-quality content.
A Comparative Analysis of Use Cases: Conversational vs Generative AI
The use cases of conversational and Generative AI Solutions are vast across different industrial sectors. Let’s compare the use cases of these AI types in detail;
Telecommunication
Conversational AI is now found globally in customer service sectors. It has replaced manual conversation with faster and automated routine queries handling and real-time responses.
Generative AI generates content for customer support, like tailored responses or FAQs beyond basic interactions.
E-commerce
Conversational AI accelerates personalized user experiences by guiding them through products and addressing inquiries within the store.
Generative AI delivers personalized buying recommendations, promotional content, and product descriptions based on user behavior.
Healthcare
Conversational AI assists with patient interactions, managing clinical service booking appointments, and delivering basic medical information.
Meanwhile, Generative AI assists in preparing patient reports, aids in research findings, and creates treatment plans.
Education
Conversational AI offers interactive tutoring and student assistance. Generative AI can develop educational content, such as customized learning materials, quizzes, or even simulations for more tailored learning experiences.
Entertainment
Conversational AI powers interactive voice assistants for music and media recommendations.
Generative AI, however, creates new music, video scripts, or game content, generating fresh creative works.
Digital Marketing
Conversational AI handles and engages customers well through personalized messaging such as chatbots.
Generative AI creates tailored marketing materials, including ads, blog posts, or social media content, improving content strategy.
Conversational AI or Gen AI – Which One to Go With?
Get a deeper idea into What is Conversational AIand Generative AI?Wondering when to employ them? Keep in mind that both AI solutions will be advantageous for your organization. However, based on your AI requirements, you can invest in any of the preferred ones.
Both Gen AI and Conversational AI use NLP – natural language processing in order to produce human-sounding content and responses. However, the Conversational AI model is purely focused on customer assistance and chatbots. It will assist your businesses in interacting with your customers in real time. It will also give quick responses to user queries around the clock. This AI technology will boost your customer support efficiency and enhance satisfaction by offering immediate help.
However, Generative AI will help your business comprehensively with the production of fresh and original content. It is especially useful when you want to develop conceptual art or generate different forms of content ideas during the planning phase. Also, in the executing phase, the Gen AI technology will provide assistance with brainstorming and creative concepts, streamlining the entire process.
Later, the AI model will also support you in making necessary changes in your content and planning based on the latest feedback and reviews from your customers. To let your business enjoy this efficiency, you need to invest in a reputed generative AI development company that will help you build your desired model.
Explore our case study to see how we’ve created a platform that turns your furry friends into breathtaking digital masterpieces.
Read the Case Study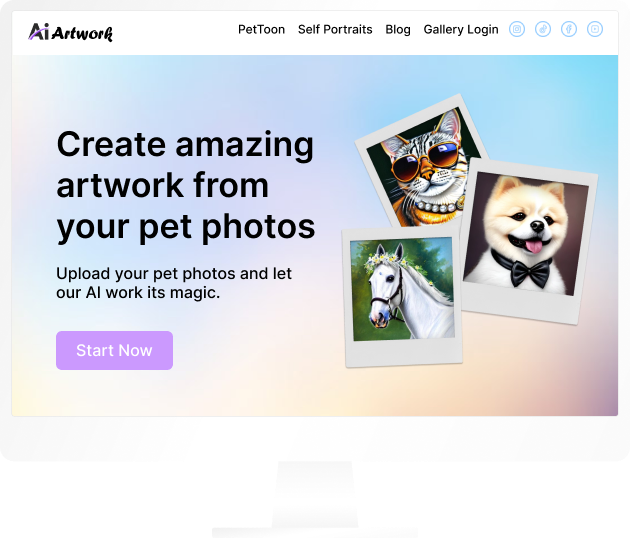
How to Implement Generative AI & Conversational AI
1. Steps to Implement Generative AI
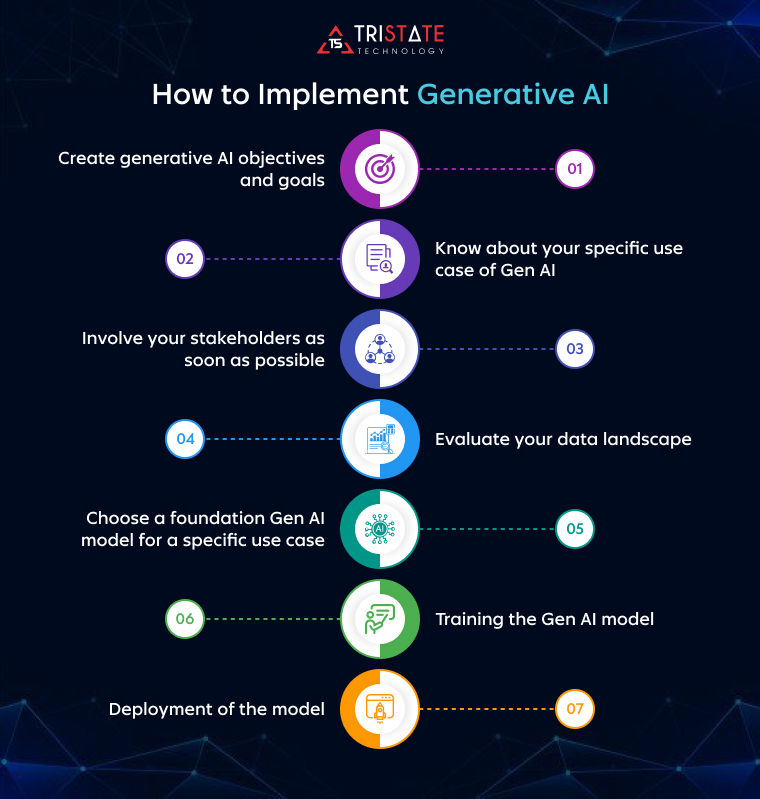
Step 1: Create generative AI objectives and goals
You need to plan and prepare the specific objectives and goals for the generative AI model to match with business needs and growth.
Step 2: Know about your specific use case of Gen AI
Clearly outline the services you want to automate and the problem you want to solve with the Gen AI model.
Step 3: Involve your stakeholders as soon as possible
You need to engage your in-house team and stakeholders early to align their expectations and gather views and insights.
Step 4: Evaluate your data landscape
Evaluate your existing data for quality, availability, and relevance to ensure the AI model is programmed effectively with them.
Step 5: Choose a foundation Gen AI model for a specific use case
Better go for a pre-trained model as a demo suited to your specific usage, considering performance and adaptability.
Step 6: Training the Gen AI model
Step 7: Deployment of the model
Next, move ahead to integrate a trained AI model into your systems for real-world applications.
2. Steps to implement Conversational AI
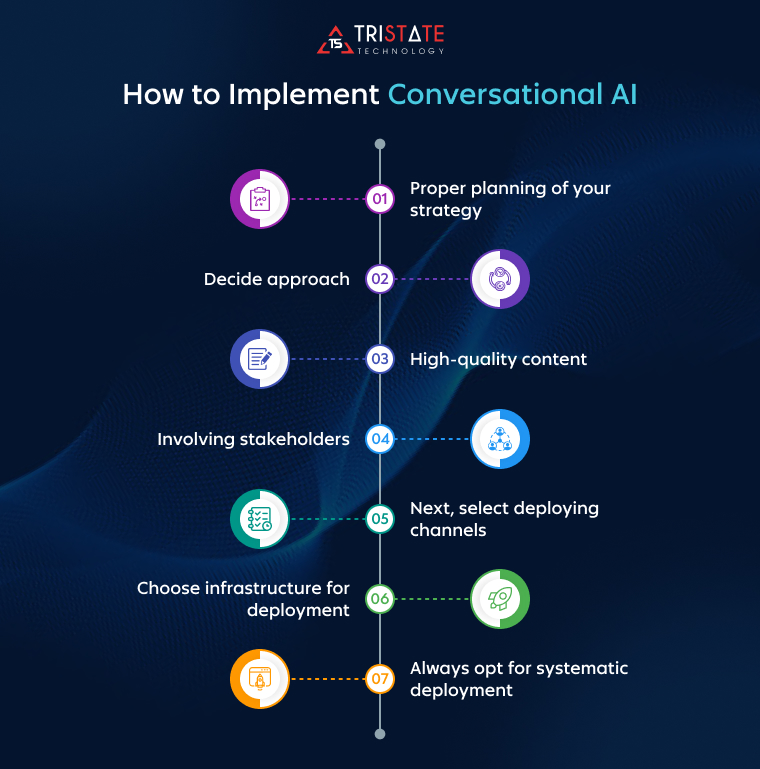
Step 1: Proper planning of your strategy
Begin by setting clear end goals and identifying how conversational AI can support your business requirements. You need to outline specific customer challenges to address them with this AI model. Define KPIs to supervise the goals.
Step 2: Decide approach
You need to figure out whether to develop your conversational AI internally or you can go with third-party platforms. Also, you can choose a conversational AI service provider.
Step 3: High-quality content
Prefer using your existing data, such as customer guidance materials and FAQs, to improve interactions with the conversational AI model. Refine this content and run roleplay demo sessions in order to create scripts and detect unexpected scenarios.
Step 4: Involving stakeholders
Do not forget to engage your security and legal teams earlier to approve the model. You must secure content approval from these teams to avoid delays in implementation.
Step 5: Next, select deploying channels
your Conversational AI use case to choose the right channels. Opt for digital channels that match your business model. Ensure that your conversational AI is tested rigorously to improve customer outcomes by providing relevant and effective interactions across the selected channels.
Step 6: Choose infrastructure for deployment
Confirm which infrastructure you want to deploy the AI on, for example, the cloud or hybrid. Each of the infrastructures comes with distinct merits and drawbacks.
Step 7: Always opt for systematic deployment
Work with your team and provider to define specifications, implementation needs, and KPIs. Launch the AI model internally for user and security testing before releasing it to customers while continuously monitoring customer behavior for feedback and potential improvements.
A Brief on Technology and Tools: Conversational AI Vs Generative AI
1. Conversational AI
- Technologies: Conversational AI solutions depend on Natural Language Processing (NLP), Natural Language Understanding (NLU), and voice and speech recognition for interactive and transparent communication.
- Tools: Popular tools for conversational AI include AI chatbots such as Drift, Intercom, Cleverbot, and Dialogflow. Voice assistant tools are Google Assistant and Amazon Alexa. AI customer service platforms are Zendesk, Freshdesk, Tidio, Qualaroo, and LivePerson.
2. Generative AI
- Technology: Gen AI uses the latest technologies like NLP, Machine Learning, GANs, and transformer models.
- Tools: Leading generative AI tools are OpenAI’s GPT-3, DALL·E, RunwayML, ChatGPT, GitHub Copilot, Character.ai, Design generators, Jasper AI, Inc and Stable Diffusion.
Key differences are in their purposes, such as conversational AI, which focuses on communication, and generative AI, which focuses on new content. Also, the difference lies in their capabilities – Conversational AI excels at user interaction and engagement, whereas generative AI masters in content creation and problem-solving. Know about the AI models in detail by finding a professional AI app development company.
Collaborate with TriState Technology to integrate the ideal AI model into your business!
Hope you got a deep understanding of the differences between Gen AI and Conversational AI. Suppose you want to develop a specific AI model for your business and partner with TriState Technology to explore the most suitable AI solutions for your business’s operational processes.
The company has expertise in the seamless implementation of AI models with custom solutions. Get in Touch with TriState Technology, to unlock the best AI strategy for business growth and take advantage of the full potential of AI to bring innovation.