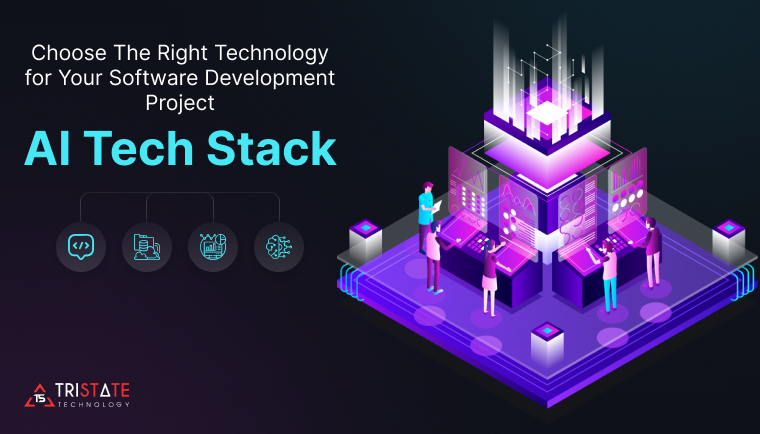
Artificial Intelligence (AI) is a powerful driver of alteration across industries today, and it has started to redesign how businesses engage with technology. Organizations from many domains are increasingly implementing AI-powered solutions to streamline developments, systematize tasks, and tailor facilities for clients. Nonetheless, the success of an AI-driven software development initiative will depend on the selection of the AI development tech stack in 2025.
Based on the abundance of AI software development tools present in 2025, it is highly likely that picking the best tech stack for AI will be challenging. An industry research report suggests that more than 85% of AI-powered software projects name scalability and integration as defining features of the tech stack that they choose.
The selection process entails an assessment of the available programming languages, AI frameworks, data processing tools, and deployment platforms about the project’s goals. The balance between scalability, performance, and cost-effectiveness is what determines the robustness of the AI-driven application.
Additionally, working with seasoned AI professionals enables firms to streamline AI model development and deployment, resulting in speedier invention and a competitive advantage. Companies can use AI Development Services to improve workflow efficiency, optimize AI model performance, and speed the implementation of cutting-edge AI solutions. This strategic strategy not only shortens development time but also increases scalability, allowing firms to respond rapidly to changing market demands.
This guide is a recommendation on AI tech stacks, their components, and the most effective approaches to consider when choosing a technology stack for your AI software project.
Table of Contents
Why the Right AI Tech Stack is Important for Your Software Project?
Choosing the right selection of AI development tech stack in 2025 has a decisive impact on project effectiveness, scalability, and overall success. A properly oriented AI tech stack enables integrating different technologies with ease, increases their performance, and saves development resources. Conversely, if the decision is not right, there will be mismanagement, incompatibility, and failure to expand the project.
Businesses that integrate well-structured AI tech stacks involve noteworthy enhancements in efficiency and cost reduction. Indicating the right AI Development Services ensures unified implementation, optimized model training, and enhanced decision-making capabilities. This strategic approach helps companies maximize productivity, streamline workflows, and stay competitive in the evolving AI landscape.
Whether it is an AI chatbot, a recommendation engine, a predictive analytics application, or a computer vision application, the right AI-powered tech stack supports the achievement of key objectives in these software projects. The selection criteria must include performance, ease of integration, ease of development, and scalability potential.
Moreover, the best tech stack for AI determines how easily the AI applications will be incorporated into everyday business operations and therefore how effective these processes will be. When an optimized stack is selected, organizations incur less technical debt, deploy faster, increase model accuracy, and enhance decision-making, customer experience, and overall satisfaction.
Understanding the AI Tech Stack
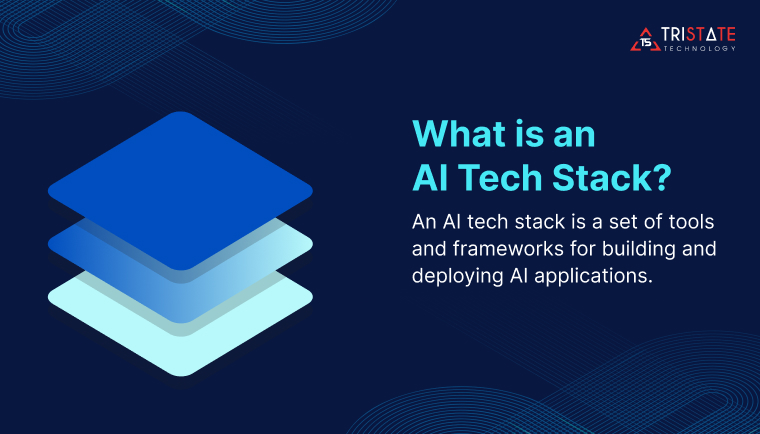
An AI tech stack is a collection of different tools and components, including programming languages, software frameworks, libraries, data management tools, DevOps tools, and frontend/backend development technologies, that are needed to create, deploy, and maintain AI-powered software applications. Each stack layer contributes towards building AI models and deploying them efficiently.
Having a well-defined AI tech stack helps in solving challenges related to development processes, while also increasing the effectiveness with which AI models can operate in the real world. Through accurate tools and technologies, industries can advance performance, diminish development time, and ensure proper system compatibility.
Furthermore, an optimized AI development tech stack encourages developers to adopt new algorithms and strategies by improving automation and model refinement infrastructure. This allows for better model accuracy and faster deployment cycles, ensuring that organizations maintain a competitive edge in AI.
More so, the addition of sophisticated AI software development tools increases the level of automation, which diminishes the amount of manual work needed, streamlining the development processes so that productivity can be maximized and AI solution delivery is enhanced.
Furthermore, adopting a well-optimized AI tech stack enables organizations to handle large datasets efficiently, improving the accuracy and reliability of AI models. By leveraging the right amalgamation of AI software development tools, companies can ensure seamless collaboration among data scientists, engineers, and developers, ultimately leading to the faster deployment of AI-driven innovations.
Additionally, choosing the best programming languages for AI development ensures that businesses can build scalable and high-performance AI applications. Using languages like Python, R, and Java, companies can create robust AI models while maintaining flexibility and ease of implementation.
The right AI tech stack components play a critical role in ensuring efficiency and meeting industry standards, making it easier for businesses to integrate AI-driven solutions into their workflows.
Understanding the Components of an AI Tech Stack
For companies seeking adequately functional and scalable AI solutions, thoughtfully selecting an AI tech stack is of utmost importance. By adding modern AI Development Services, organizations can improve the training, deployment, and monitoring of models to ensure accurate functionality and better decision-making.
Furthermore, picking tools with strong documentation and industry adoption helps in long-term upkeep and troubleshooting. By ranking these key considerations, organizations can develop AI solutions that deliver optimal results and long-term value.
With the right AI tech stack, businesses can advance efficiency, reduce operational costs, and stay in advance in the competitive AI countryside. A well-structured AI environment ensures seamless partnership between development teams and fosters innovation.
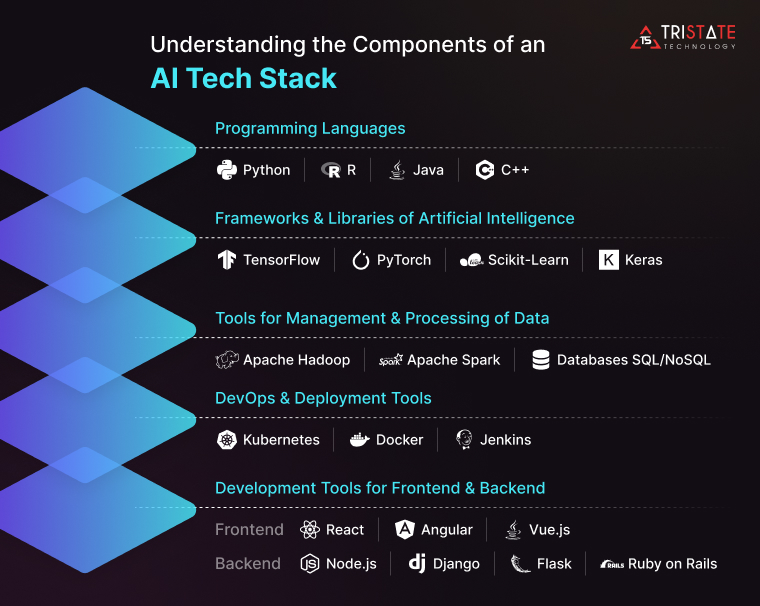
1. Programming Languages
One of the most significant choices in AI development is programming language selection.
Here are some of the additional current picks:
- Python: Along with being the most popular language in AI and machine learning, it offers an abundance of libraries and has a supportive community.
- R: Best for performing data analysis and statistical computing.
- Java: Provides great capabilities for scalability, performance, and robust applications.
- C++: Known to be more powerful and effective for the seamless execution of high-level applications.
2. Frameworks and Libraries of Artificial Intelligence
The implementation of machine learning algorithms is made easier through the use of different AI frameworks. Here is a list of some of the most widely used frameworks and libraries:
- TensorFlow: An open-source deep erudition framework known for its flexibility and all-embracing ecosystem. It is extensively used for training and organizing machine learning mockups at scale.
- PyTorch: PyTorch is identified for its dynamic computation graph, making it easier to debug and research with neural networks. It is a favored choice for AI research and development.
- Scikit-Learn: A machine learning library for Python that delivers simple and well-organized tools for data preprocessing, classification, regression, clustering, and more.
- Keras: A sophisticated API created on topmost of TensorFlow that abbreviates neural network development, making it available for beginners while still being powerful enough for intricate applications.
3. Tools for Management and Processing of Data
Numerous software applications that incorporate AI technologies have large datasets that need efficient processing and storage tools:
- Apache Hadoop: This framework facilitates the distributed storage and processing of large sets of data. It is extensively used in big data applications, serving businesses to manage and examine vast amounts of information professionally.
- Apache Spark: This framework offers fast in-memory computing, specifically used to process big data.
- Databases SQL/NoSQL: This subsumes within it all databases such as MySQL, PostgreSQL (SQL) along MongoDB, and Cassandra (NoSQL) which are used for both structured and unstructured data. These databases help accomplish vast quantities of information competently, ensuring scalability and high performance for AI-driven applications.
4. DevOps and Deployment Tools
The deployment of AI models needs seamless integration with tools of continuous operations that focus on scaling and monitoring:
- Kubernetes: Automates the deployment of containerized applications and enables AI engineering at scale. It allows efficient orchestration, seamless updates, and resource optimization for AI assignments.
- Docker: Facilitates the deployment of applications by encapsulating the application along with supporting software. This guarantees constancy across numerous environments, making the deployment process more well-organized and reducing potential compatibility issues.
- Jenkins: Implements automation of the integration and deployment phases of development through CI/CD pipelines. This enhances workflow effectiveness by reducing manual intervention and minimizing errors in AI application releases.
5. Development Tools for Frontend and Backend
AI-driven applications need a seamless user interface.
- Frontend development frameworks include React, Angular, and Vue.js for building user interfaces.
- Backend programming frameworks are Node.js, Django, Flask, and Ruby on Rails for processing application logic and API calls.
Types of AI Tech Stacks Based on Project Needs
Knowing how to choose an AI tech stack is vital for ensuring maximum efficiency and scalability. The optimal AI stack selection relies heavily on the specifics of your project. Getting the right tools is essential for success, whether you’re dealing with data, NLP, computer vision, or AI applications.
Selecting the right AI tech stack for a project depends on various influences such as data complexity, computational needs, and scalability necessities. Each AI application has exclusive challenges, and the choice of programming languages, frameworks, and deployment tools significantly impacts its success. Businesses must prudently assess their requirements and align them with the most appropriate technology stack to ensure efficiency, flexibility, and long-term sustainability.
Different AI projects require certain specific tech stacks tailored to suit their parameters:
- Machine Learning and Data Science Projects: These require Python, Jupyter Notebooks, TensorFlow, and Apache Spark. Python is one of the greatest widespread AI programming languages. Jupyter is used as an interactive programming environment. TensorFlow is extensively used for deep learning, while Apache Spark is employed in large-scale data processing.
- Natural Language Processing (NLP) Applications: These projects utilize SpaCy and NLTK, which are NLP-specific libraries used for human language data processing and analysis.
- Computer Vision Projects: OpenCV image processing library, as well as TensorFlow and PyTorch, are the primary dependencies. Both TensorFlow and PyTorch are extensively used for deep learning model training in image recognition and object detection.
- AI-Based Web and Mobile Development: These use traditional software frameworks with incorporated AI capabilities. AI libraries and web/mobile development languages with frameworks, such as React, Angular, and Flutter, are also needed alongside the TensorFlow.js web AI library.
Stages of the Modern AI Tech Stack
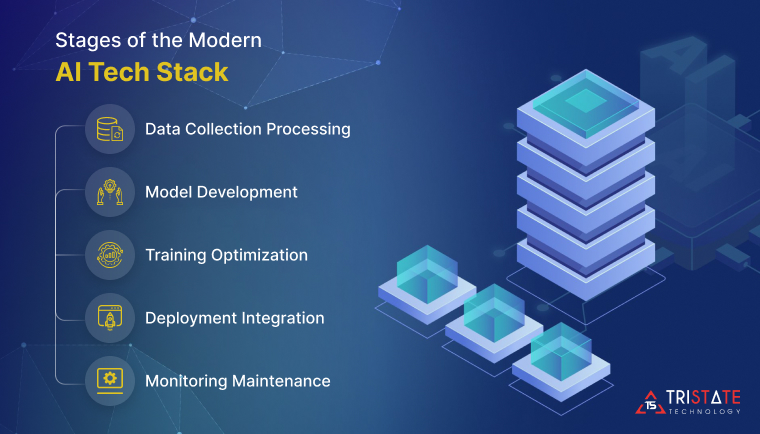
Each phase of an AI tech stack is integral to supporting the construction of an error-free and scalable AI system. The entire process, from model monitoring to data collection, requires the incorporation of different AI software development tools.
These phases help ensure that AI systems function properly, change with new data, and give accurate outputs. Knowing these steps allows organizations to automate processes and improve AI-based decision-making.
By deliberately aligning AI software development tools with project necessities, businesses can optimize workflows, boost scalability, and improve overall performance. A well-structured implementation roadmap is key to building a maintainable and future-proof AI-driven system.
1. Data Collection Processing
Incorporates the processes of collecting, saving, and manipulating data using ETL tools, databases, and data lakes. Actual data handling ensures seamless incorporation, faster processing, and better-quality model accuracy.
2. Model Development
Involves developing and experimenting with AI models on infrastructure with the available AI frameworks and machine learning services. This phase safeguards that the selected technologies align with the project’s objectives, optimizing presentation and scalability.
3. Training Optimization
Pacts with fine-tuning the algorithms as well as the act of the model. Guaranteeing the greatest model demonstration is pivotal for real-world applications.
4. Deployment Integration
Takes care of deploying several AI model issues on different clouds and managing them using various DevOps tools. These tools assist in streamlining model apprises, automating workflows, and improving operational efficiency for AI-driven applications.
5. Monitoring Maintenance
Safeguards incessant learning, updates, performance optimization, and seamless AI model version to evolving data patterns, enabling businesses to uphold high efficiency and accuracy in AI-driven applications.
Key Factors to Consider When Choosing an AI Tech Stack
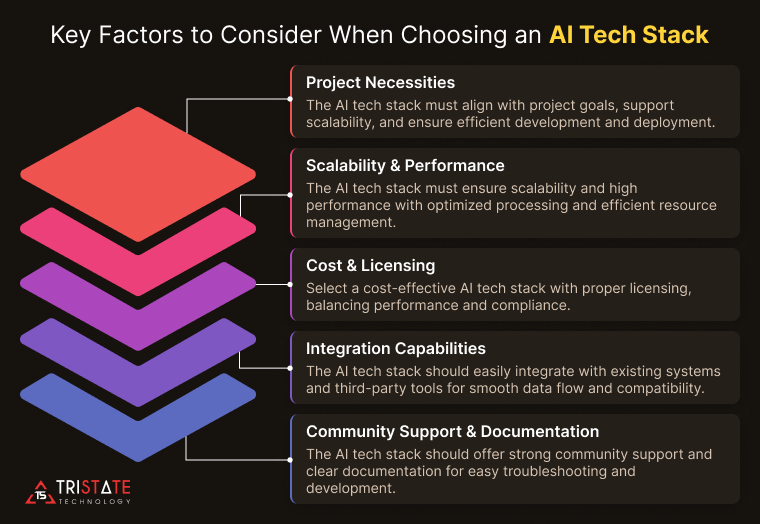
Indicating the precise AI tech stack needs a cautious valuation of numerous factors to ensure compatibility, competence, and scalability. Businesses should analyze their project’s complexity, future growth prospects, and budget constraints. A well-structured AI tech stack must integrate seamlessly with existing systems, provision high-performance computing, and offer robust community support.
Additionally, selecting tools with robust documentation and industry adoption helps in long-term maintenance and troubleshooting. By prioritizing these key considerations, organizations can develop AI solutions that deliver optimal results and long-term value.
- Project Necessities: Clearly outline the goals and technical needs of your AI application to determine the necessary tools and technologies.
- Scalability & Performance: Confirm that the AI tech stack can handle cumulative workloads and uphold competence as the project grows.
- Cost & Licensing: Comparison of open-source and proprietary AI software development tools to balance affordability with functionality.
- Integration Capabilities: Select a tech stack that works well with your existing infrastructure to minimize compatibility issues.
- Community Support & Documentation: Opt for tools with all-embracing documentation and active developer societies to facilitate troubleshooting and ongoing developments.
An All-inclusive Step-by-Step Guide to Selecting the Right AI Tech Stack
Choosing the ideal AI tech stack needs a strategic method to ensure competence and scalability. Start by defining the exact goals of your AI development, whether it’s machine learning, natural language dispensation, or data analytics. Appraise the compatibility of different AI tech stack components with programming languages, AI frameworks, and data processing tools with your project requirements.
Additionally, deliberate the long-term sustainability of the selected stack by analyzing its community support, integration capabilities, and performance benchmarks. Consulting with industry experts or partnering with the best AI development company can further modernize the decision-making process and help you influence the most advanced AI development services accessible.
- Establish the aims and parameters of your project.
- Recognize the use of case-based components of the AI tech stack.
- An analysis of AI frameworks and programming languages.
- Estimate your needs for data processing and storage.
- Choice of the tools for deployment and monitoring.
- Think about future maintenance and scalability.
- For insights, speak with the top AI development firm or specialists in the field.
Thorough Analysis and Contrast of Leading AI Tech Stacks
Indicating the exact AI technology stack can have a big effect on the achievement of your project. Different AI stacks have discrete features, performance capabilities, and compatibility factors. Understanding their strengths and ideal use cases enables organizations to make informed judgments.
Here’s an evaluation of some of the most prominent AI tech stacks for 2025.
AI Tech Stack | Key Tools | Benefits | Best Use Cases |
TensorFlow Stack | Python, TensorFlow, Keras | Strong community, deep learning capabilities | Image recognition, NLP, AI research |
PyTorch Stack | Python, PyTorch, NumPy | Dynamic computation graph, easy debugging | Research, AI startups, deep learning |
Scikit-Learn Stack | Python, Scikit-Learn, Pandas | Simple and effective for traditional ML | Basic ML projects, data science |
Apache Spark Stack | Scala, Spark MLlib, Hadoop | Handles large datasets, distributed computing | Big data AI applications |
Operative Strategies to Enhance Your Existing AI Tech Stack
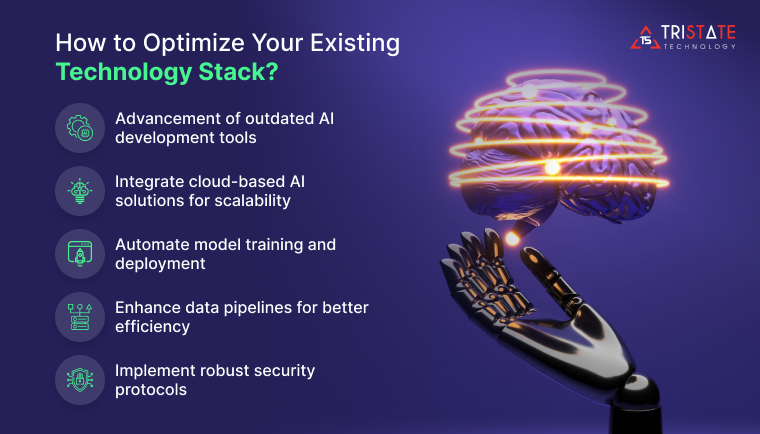
Enhancing an AI tech stack needs incessant evaluation and development to enhance performance and competence. Industries leveraging AI development services can streamline procedures, advance model accuracy, and guarantee seamless incorporation with developing technologies. Regular updates, automation of workflows, and cloud-based solutions contribute to making AI implementations more scalable and cost-effective.
Also, AI integration automates processes, taking pain off developers’ shoulders by managing workflow and reducing mistakes. Organizations adopting Machine Learning Services can improve their predictive analytics, decision-making capabilities, and innovative processes.
Companies can maintain model effectiveness and flexibility while undergoing technological changes by improving their AI infrastructure.
- 1. Advancement of outdated AI development tools.
- 2. Integrate cloud-based AI solutions for scalability.
- 3. Automate model training and deployment.
- 4. Enhance data pipelines for better efficiency.
- 5. Implement robust security protocols.
Pro Tips for Picking the Best AI Tech Stack
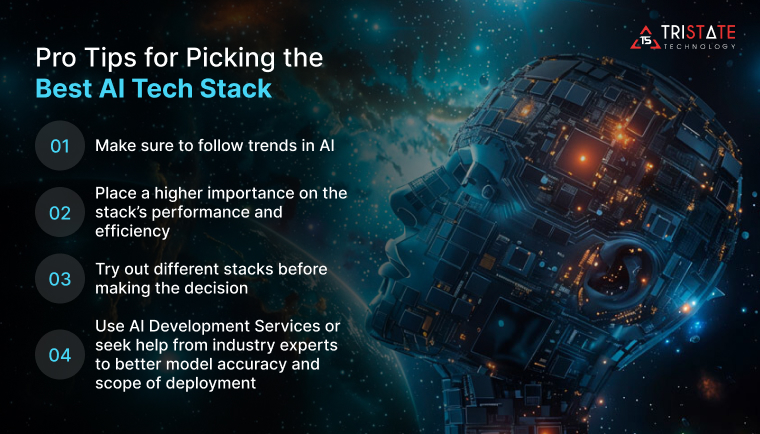
While choosing the best AI tech stack, companies should consider elements such as scalability, integration, and practicality. With the help of AI Development Services, the selection can be simplified through expert assistance and offers of the most recent innovations in AI technologies.
A study shows that 72% of companies that incorporated AI development services reported a considerable increase in operational efficiency. Companies can fine-tune their AI solutions to business demands and market changes by working with the appropriate service providers.
Furthermore, working with the Best AI Development Company safeguards access to top-tier expertise, helping businesses deploy AI-driven applications efficiently and maintain a competitive edge in the market.
- Make sure to follow trends in AI.
- Place a higher importance on the stack’s performance and efficiency.
- Try out different stacks before making the decision.
- Use AI Development Services or seek help from industry experts to better model accuracy and scope of deployment.
Our experts help you select and implement the right AI tech stack to align with your business goals and technical roadmap.
Speak to an AI Consultant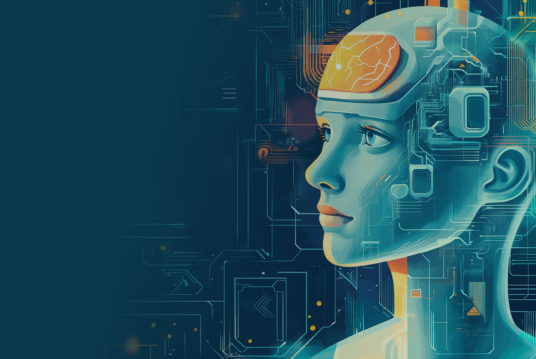
Final Thoughts
Selecting a suitable tech stack for the development of AI projects is very important. Knowing the components of the AI tech stack, evaluating the critical factors, and using a methodical approach will aid decision-making. In the case that you are developing a machine learning model, an NLP application, or an AI web solution, you can rest assured that having the right AI development tech stack 2025 will help in productivity, scalability, and creativity with your AI projects.
Working with the best AI development company will further improve the outcome of one’s project because they provide expert knowledge, tailored solutions, and effortless deployment. Using machine learning services guarantees better automation, effective AI software, and smart decision-making for improved predictive outcomes.
When businesses invest in the right AI tech stack, they set the foundation for seamless integration and superior performance of their AI applications. The excellent programming languages, frameworks, and data management tools directly impact the speed and correctness of model training, deployment, and execution.
Partnering with the Best AI Development Company can simplify this decision-making process, guaranteeing that organizations adopt cutting-edge technologies tailored to their exact needs.
Additionally, taking the right AI stack permits industries to stay in advance of business trends and technological developments. By capitalizing on scalable and high-performance AI development services, organizations can streamline operations, enhance customer experiences, and drive innovation. Leveraging cloud-based AI explanations and automation tools can augment scalability, minimize infrastructure costs, and optimize computational competence. The ability to adapt AI models to altering business demands is crucial, making the selection of an optimized tech stack a key determinant of project success.